Introduction
The KB Europeana Newspapers NER dataset was created for the purpose of evaluation and training of NER (named entities recognition) software. The original OCR of a selection of European newspapers has been manually annotated with named entities information to provide a 'perfect' result, otherwise also known as ground truth. The collections of four project partners has been manually tagged with all instances of Person, Location and Organisation and this data is avilable in CC0 via this Lab.
There are four sets available for download, namely;
- Dutch from the Koninklijke Bibliotheek
- Austrian from the National Library of Austria
- German from the Dr. Friedrich Teßmann Library
- French from the National Library of France, in cooperation with LIP6-ACASA
Each set consist of a number of ALTO files, a BIO file and a trained classifier for Stanford NER.
When using this dataset we request you to cite it as follows:
Europeana Newspapers project, (2014), KB Europeana Newspapers NER Dataset. KB Lab: The Hague. http://lab.kb.nl/dataset/europeana-newspapers-ner
Access
Each set can be downloaded in Zip-files and consist of ALTO files, a BIO file and a trained classifier.
Named Entities in Dutch Newspapers Set:
- ALTO (md5sum: d481d7e40d84bb479a479aa5266f8d0d)
- BIO (md5sum: 4724261eca17dc7eb7daad6175627488)
- Trained classifier for Stanford NER: eunews.nl.crf.gz (153 MB)
Named Entities in German Newspapers Set:
- ALTO (md5sum: 217f955f9c7e643c99c4657611bb3570)
- BIO (md5sum: 85872b4841fbaec49268839cdd0875a4)
- Trained classifier for Stanford NER: eunews.de.crf.gz (19 MB)
Named Entities in Austria Newspapers Set:
- ALTO (md5sum: 3b4e76e268f64ed26956b4a677e8043e)
- BIO (md5sum: 3d0e909baf9aedfc27673170ed1fcdff)
- Trained classifier for Stanford NER: eunews.at.crf.gz (34 MB)
Named Entities in French Newspapers Set:
- BIO (md5sum: cf8d59c45bca837fc076eae403fcd166)
- Trained classifier for Stanford NER: eunews.fr.crf.gz (47 MB)
Examples
All methods have been evaluated by retaining a number of pages per language for evaluation. Precision and Recall are calculated using the amount of true positives, true negatives, false positives and false negatives.
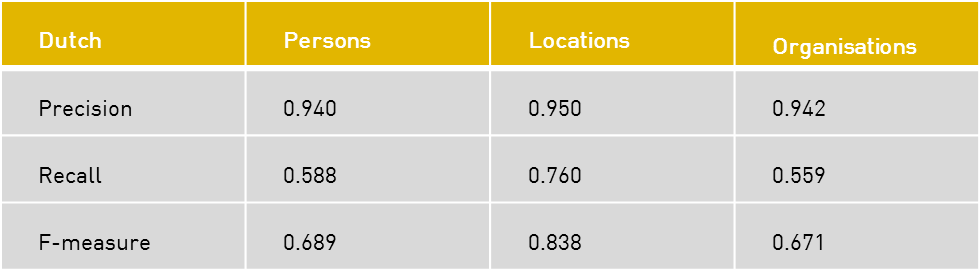
These figures have been derived from a k-fold cross-evaluation of 25 out of 100 manually tagged pages of Dutch newspapers from the KB. The results confirm the fact that the Stanford NER tagger tends to be a bit “conservative”, i.e. it has a somewhat lower recall for the benefit of higher precision, which is also what was aimed for, as this is the most valuable for our users.
The French material was evaluated by LIP6, which resulted in the following figures:
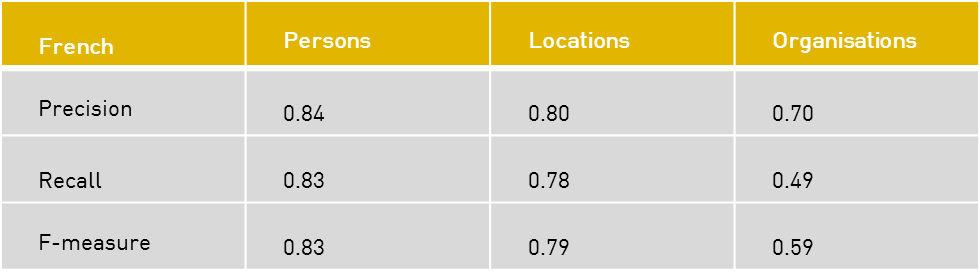
There were less pages available for evaluation for German and Austrian, due to the problems with the export function of the training tool that resulted in several pages being not useable. The decision was made to use as many as possible for training, which resulted in a smaller evaluation set. Therefore, the outcomes are not split up per category, as this would provide too little entities for a good evaluation. Five pages from LFT and six pages from the ONB were used for the following evaluation.
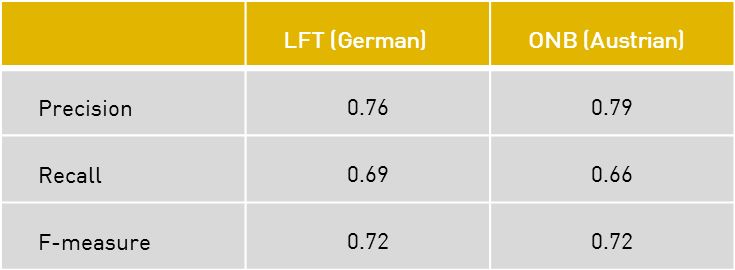