The KB has been digitising its newspaper collection since 2006 and has now published more than 12 million pages on the national platform Delpher.nl. Over these past 12 years optical character recognition (OCR) has improved a great deal – partly due to the efforts made in the by the KB coordinated FP7 project IMPACT.
OCR quality at the KB
However, we have never really known what the quality is of the OCR we provide to our users and more importantly perhaps, we would like to improve the older OCR. We have therefore started a project where we intend to find out what the quality is of our newspaper OCR and if there is a (semi-)automated process we can use to improve it. We will be looking at reOCRing old files, a machine learning application (Ochre by dr. Janneke van der Zwaan) and a text induced corpus cleaning application (TICCL by dr. Martin Reynaert). We also did a small test to see what the difference was between OCRing from master or access images and found that the difference wasn't actually significant!
Project setup
To evaluate the OCR we need ground-truth (99,95% corrected text) and this is a rather costly process. This is why we have chosen to work with a sample of our newspapers of 2000 pages – a mere 0,17% of the total. We know this is very little, but we do hope this gives us some insights into what we have and which options we have to improve it without it costing us an arm and a leg (which could be used for more digitisation).
OCR metadata
To select a representative sample we first needed an overview of the total amount of pages and with which software package there were processed. This is all information that is available in the ALTO files of the digitised newspapers, in the tag <OCRProcessing>:
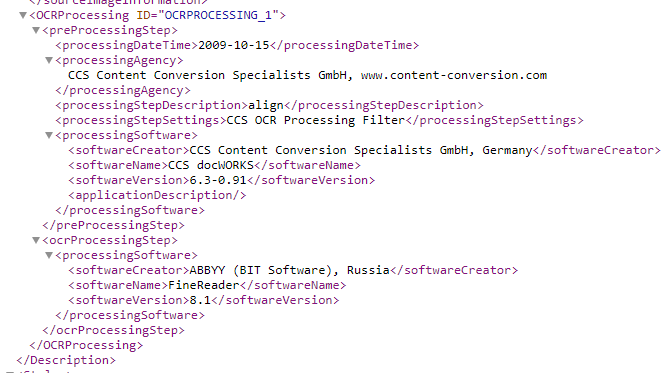
Image 1: OCRProcessing tag in KB's ALTO files
In February 2017 we extracted all information from the ALTO files we had online at that time and after some cleaning using OpenRefine inputted them into a database (available on request). That resulted in the following overview. All our newspapers are digitised with ABBYY software and the versions ranged from 7.0 to 10.0.
One discrepancy was found in the data and that were a number of files that had both ABBYY 8.1 and 9.0 as processing software in their metadata. We could not find a clear explanation for this and have therefore decided to mark all these files as 8.1.
Selection of sample
Next to the division on software versions, we also chose to divide the data in time slots looking at spelling changes in Dutch. We also opted to exclude all 17th century newspapers, because of their bad OCR and the fact that they are being manually corrected in a volunteer project. This is also the case for a large selection of WW2 newspapers, which have therefore also been excluded. This resulted in the following selection of pages (with some deviations due to rounding off):
Years/software | ABBYY 8.1 | ABBYY 9.0 | ABBYY 10.0 | Total |
1700-1882 | 237 | 0 | 37 | 275 |
1883-1947 (minus 1940-1945) | 652 | 21 | 494 | 1166 |
1948-1995 | 436 | 11 | 112 | 559 |
Total | 1325 | 32 | 643 | 2000 |
Retrieving images
As one of our test strands is assessing what reOCRing old files yields in improvement, we also needed to select the images we would be using in our sample. When digitising we have two types of images delivered, one for access purposes and one for preservation purposes. Both are JPEG2000, but the preservation copy (master image) is lossless while the access copy is lossy. A small selection of the master images is also in greyscale. Harvesting the access images is easy, we can simply use our API for this. However, accessing the master images requires a lot more work, as they are stored on tape and we would need to use a specific application to retrieve the batches that is also used for other purposes and can currently only handle one process at a time.
Master or access images
We thus wanted to know if we could use access images in our project instead of master images. We therefore conducted a small test of 23 pages and had the master and access images reOCRed with ABBYY FineReader 11 and the ALTO files groundtruthed. We used a similar division for the selection of these files as in the table above. The IMPACT Centre of Competence then evaluated the files for us (which is a service they provide to us as member) using their evaluation tool, resulting in the following outcomes:
Word error rate (independent word order) |
|||
Document |
Original |
Access |
Master |
DDD_010335677_009_alto |
15,21 |
9,76 |
9,01 |
DDD_010336697_007_alto |
3,32 |
3,24 |
2,67 |
DDD_010378395_001_alto |
2,27 |
2,16 |
1,95 |
DDD_010383104_010_alto |
32,81 |
31,75 |
29,98 |
DDD_010383609_009_alto |
3,97 |
4,95 |
4,29 |
DDD_010386076_005_alto |
11,42 |
8,40 |
7,48 |
DDD_010391633_001_alto |
36,35 |
35,45 |
32,10 |
DDD_010392330_002_alto |
11,49 |
7,12 |
6,92 |
DDD_010393345_004_alto |
17,71 |
14,45 |
14,22 |
DDD_010415304_001_alto |
27,11 |
16,79 |
15,86 |
DDD_010415398_004_alto |
17,37 |
13,94 |
12,41 |
DDD_010416785_003_alto |
14,85 |
10,97 |
10,50 |
DDD_010418067_007_alto |
4,59 |
4,21 |
3,87 |
DDD_010459584_015_alto |
2,15 |
1,74 |
1,55 |
DDD_010459584_030_alto |
2,66 |
0,72 |
0,66 |
DDD_010459586_001_alto |
13,18 |
12,95 |
12,74 |
MMGAVL01_000000601_004_alto |
5,89 |
5,80 |
5,69 |
MMGAVL01_000005301_001_alto |
3,00 |
4,23 |
4,18 |
MMGAVL01_000009309_006_alto |
9,58 |
6,61 |
6,91 |
MMGAVL01_000011666_002_alto |
4,71 |
5,33 |
5,45 |
MMGAVL01_000014994_007_alto |
5,17 |
7,08 |
6,75 |
MMGAVL01_000038349_003_alto |
9,05 |
10,93 |
10,41 |
MMGAVL01_000039711_001_alto |
2,16 |
1,44 |
1,61 |
Average |
11,13 |
9,57 |
9,01 |
P-value access vs master |
0,8247925 |
You can see that the word error rate has indeed decreased (the lower the better) in most files, with the master images producing a lower number than the access images. However, when comparing them we calculated that the difference is in fact not significant in our test. We therefore chose to use access images in our reOCRing strand.
Selection files sample
Now that we knew that we could use access images and how the division of files should be between software versions and over time we could select the identifiers to be included in the sample. Using the database mentioned earlier we took a random selection of each category, resulting in a list of 2000 newspaper issues. Due to the fact that OCR for us is most relevant on newspaper articles we chose to only select the first and second page of a newspaper to ensure we would have the most articles and the least amount of advertisements. These images, OCR and metadata were then downloaded and made available to our partners in this project.
What happens next?
Over the next few months we will be working together with a service provider to get the 2000 groundtruth files ready for the evaluation. We will also provide the original ALTO files and a selection of the groundtruth files to our external partners working on the post-correction software who will use the data to train their tools and improve the files. Once we have all files corrected, the IMPACT Centre of Competence will again provide an evaluation on all files, which we will of course share here!
All data created and gathered in this project is available for reuse. Some of it contains in-copyright material, which is why we cannot publish it openly, but feel free to contact dh@kb.nl and we can provide you with a copy.